When it comes to teaching AI models to understand and use human language, two key methods come into play: RLHF and RLAIF. These might sound like complicated terms, but they’re essentially different ways to make AI smarter. Annolive plays a big part in this process by making it easier and more effective. Let’s break down what RLHF and RLAIF are and how Annolive helps in using them.
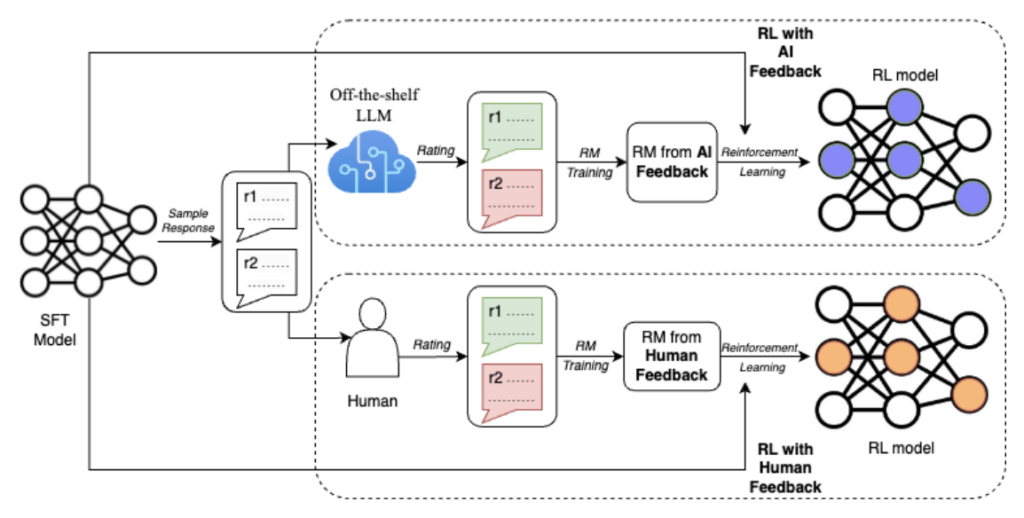
We are going to discuss the following:
- What is RLHF? Its Pros and Cons
- What is RLAIF? Its Pros and Cons
- When to Use RLHF vs RLAIF?
- How Annolive Helps with Both RLHF and RLAIF?
1. What is RLHF?
RLHF stands for Reinforcement Learning with Human Feedback. This method is like having a teacher for your AI. People review the AI’s work and give it feedback, helping it learn from its mistakes and successes. This approach is great for making AI understand things the way humans do, especially in tricky situations where understanding context or emotions is important.
Pros of RLHF:
- Human touch: By getting feedback from humans, AI can learn complexideas and nuances
- Trust: AI trained with human feedback can be more reliable and makefewer mistakes.
- Custom fit: You can make the AI learn specific things that are important for your project.
Cons of RLHF:
- Time and money: It can get expensive and slow, especially if you need experts.
- Consistency: Different people might give different feedback, which canconfuse the AI
2. What is RLAIF?
RLAIF means Reinforcement Learning with AI Feedback. Instead of people,another AI system checks the AI’s work. It was introduced in the paper : RLAIF: Scaling Reinforcement Learning from Human Feedback with AIFeedback. This is like having a robot teacher. It’s a quicker way to train AIbecause it doesn’t need to wait for people to review its work, and it canwork around the clock.
Pros of RLAIF:
- Fast: It speeds up the training process since AI can work faster than humans.
- Scalable: Good for training on a large scale because it doesn’t get tired or need breaks.
- Consistent: Feedback is more uniform because it’s coming from a programmed system, not people with different opinions.
Cons of RLAIF:
- Less depth: Might not catch the finer points or deeper understanding that human feedback could provide.
- Depends on AI quality: The success depends a lot on how good the AI teacher is.
Annolive supports the use of both publicly available models and your own proprietary models for bulk annotation. If you are using LLMs, annolive lets you to tune the prompt before annotating the whole data for RLAIF
3. When to Use RLHF vs RLAIF or Combination?
Choosing between RLHF and RLAIF depends on what you need your AI todo. If your project needs a deep understanding of human ideas or emotions,RLHF might be better. If you need to train your AI fast and on a large scale,RLAIF could be the way to go.
When to Use RLHF:
- When human touch is crucial: If the application requires understanding complex human emotions or cultural nuances, human feedback might provide better guidance.
- Scalable: Good for training on a large scale because it doesn’t get tired or need breaks.
- Consistent: Feedback is more uniform because it’s coming from a programmed system, not people with different opinions.
Cons of RLAIF:
- Less depth: Might not catch the finer points or deeper understanding that human feedback could provide.
- Depends on AI quality: The success depends a lot on how good the AI teacher is.
4. How Annolive Helps with Both RLHF and RLAIF
In Annolive you can annotate data using RLHF, RLAIF and in combination.
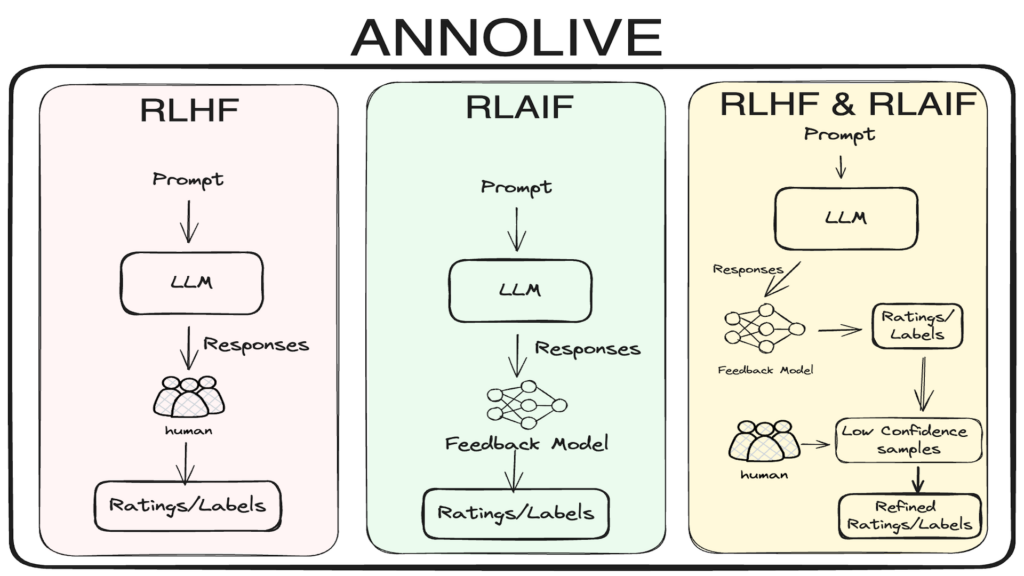
Once the bulk annotation process is completed, you can go to individual data items, if necessary, to refine the annotations. Or you can directly export the annotated dataset
References
- Bulk Annotation
- Auto Annotation
Thanks for reading. Please contact us for any queries.